Abstract Reasoning in Large Multimodal Models
Rhythm Blues AI - Podcast tekijän mukaan Andrea Viliotti, digital innovation consultant (augmented edition)
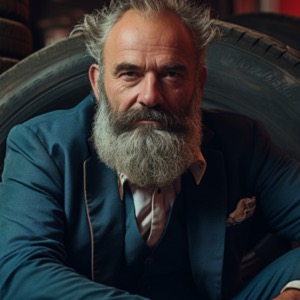
This episode provides an analysis of the capabilities of large multimodal models (MLLMs) in non-verbal abstract reasoning. The experiment employs various versions of the Raven's Progressive Matrices, a standard test for measuring fluid intelligence, to evaluate the models' ability to interpret visual relationships and deduce missing parts of puzzles based on abstract rules. The results show that open-source models underperform compared to closed-source ones, such as GPT-4V, which demonstrate significantly more advanced reasoning capabilities. The study highlights the need to develop more robust evaluation methods for these models and address their limitations, particularly their inability to accurately perceive visual details and provide reasoning consistent with visual information. Finally, the document explores the implications of these findings for the future development of MLLMs and their ethical and strategic implications for companies.