GSM-Symbolic: Understanding the Limitations of Mathematical Reasoning in Language Models
Rhythm Blues AI - Podcast tekijän mukaan Andrea Viliotti, digital innovation consultant (augmented edition)
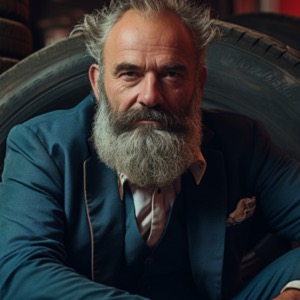
Recent advancements in artificial intelligence have led to the rise of large language models (LLMs) capable of handling complex tasks, including mathematical reasoning. A comprehensive study by Mirzadeh et al. (2024) highlighted limitations in GSM8K, a popular evaluation benchmark for LLMs in mathematics. The researchers identified issues such as data contamination, the inability to vary question complexity, and a lack of diversity in problem types. To address these limitations, they developed GSM-Symbolic, a new benchmark that allows a more accurate and flexible assessment of LLMs’ mathematical reasoning abilities. GSM-Symbolic uses symbolic templates to generate various question versions, enabling developers to test model robustness and their ability to handle different levels of complexity. The study revealed that current LLMs are highly sensitive to small changes in questions, showing structural fragility in mathematical reasoning. This underscores the need for more robust and precise models for tasks requiring logical and mathematical reasoning, emphasizing the importance of rigorous evaluation before implementing LLMs in real-world enterprise contexts.