Putting the "science" in data science: the scientific method, the null hypothesis, and p-hacking
Linear Digressions - Podcast tekijän mukaan Ben Jaffe and Katie Malone
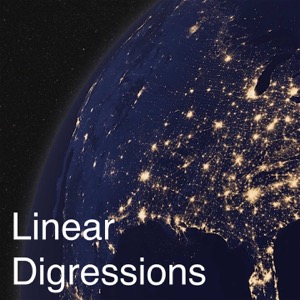
Kategoriat:
The modern scientific method is one of the greatest (perhaps the greatest?) system we have for discovering knowledge about the world. It’s no surprise then that many data scientists have found their skills in high demand in the business world, where knowing more about a market, or industry, or type of user becomes a competitive advantage. But the scientific method is built upon certain processes, and is disciplined about following them, in a way that can get swept aside in the rush to get something out the door—not the least of which is the fact that in science, sometimes a result simply doesn’t materialize, or sometimes a relationship simply isn’t there. This makes data science different than operations, or software engineering, or product design in an important way: a data scientist needs to be comfortable with finding nothing in the data for certain types of searches, and needs to be even more comfortable telling his or her boss, or boss’s boss, that an attempt to build a model or find a causal link has turned up nothing. It’s a result that often disappointing and tough to communicate, but it’s crucial to the overall credibility of the field.