Dynamic Search for Inference-Time Alignment in Diffusion Models
Best AI papers explained - Podcast tekijän mukaan Enoch H. Kang - Torstaisin
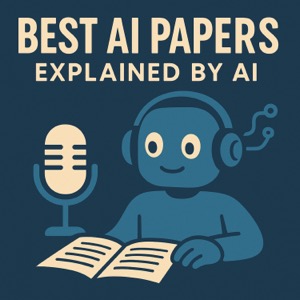
Kategoriat:
This paper highlights the challenge of aligning diffusion models with desired outcomes by optimizing reward functions, especially when gradient information is unavailable. The core contribution is the proposal of DSearch, a novel gradient-free method that reframes this alignment as a search problem on a dynamically constructed tree representing the diffusion process. DSearch utilizes heuristic functions and dynamic scheduling to efficiently explore the search space and identify high-reward samples. Experimental results across image generation, biological sequence design, and molecular optimization tasks demonstrate DSearch's effectiveness in balancing reward maximization, sample quality, and diversity.