Prediction-Powered Statistical Inference Framework
Best AI papers explained - Podcast tekijän mukaan Enoch H. Kang - Perjantaisin
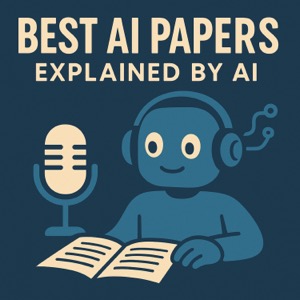
Kategoriat:
This paper presents "Prediction-Powered Inference," a novel framework for conducting statistical inference while integrating predictions from machine learning systems with experimental data. The authors, including Anastasios N. Angelopoulos, Stephen Bates, Clara Fannjiang, Michael I. Jordan, and Tijana Zrnic, propose algorithms that calculate provably valid confidence intervals for various statistical measures, like means and regression coefficients, without making assumptions about the specific machine learning model used. A key benefit is that more accurate predictions lead to narrower confidence intervals, increasing data efficiency. The framework's effectiveness is demonstrated through diverse applications, highlighting its potential to improve research conclusions across multiple scientific fields.