Q♯: Distributional RL for Optimal LLM Post-Training
Best AI papers explained - Podcast tekijän mukaan Enoch H. Kang - Torstaisin
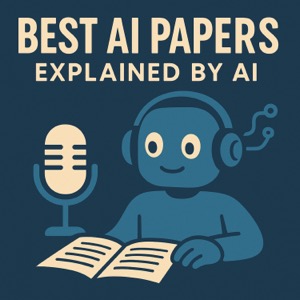
Kategoriat:
This podcast introduces Q♯, a novel reinforcement learning algorithm tailored for post-training large language models (LLMs) by utilizing distributional value functions within a KL-regularized framework. Unlike prevalent policy-based methods and existing value-based baselines that use unregularized Q-values, Q♯ learns the optimal regularized Q-function to guide the reference policy, offering theoretical guarantees and empirical advantages in math reasoning tasks while maintaining proximity to the original model. Theoretically, the work establishes a connection between KL-regularized RL and no-regret online learning, yielding variance-dependent performance bounds. Experimental results on math benchmarks and a synthetic task demonstrate Q♯'s effectiveness in improving performance and correcting pre-training biases compared to existing methods.