RM-R1: Reward Modeling as Reasoning
Best AI papers explained - Podcast tekijän mukaan Enoch H. Kang - Perjantaisin
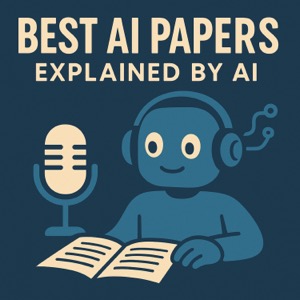
Kategoriat:
This academic paper proposes and evaluates Reasoning Reward Models (REASRMS), a novel approach to training large language models (LLMs) to align with human preferences. The core idea is to formulate reward modeling not just as assigning a score but as a reasoning task where the model generates explicit justifications and evaluation rubrics for its preference judgments. The authors introduce RM-R1, a family of REASRMS trained using a two-stage pipeline: distillation of high-quality reasoning chains followed by reinforcement learning with verifiable rewards. Empirical results show that RM-R1 models achieve state-of-the-art or near state-of-the-art performance on multiple benchmarks while offering enhanced interpretability through their generated reasoning traces and rubrics.keepSave to notecopy_alldocsAdd noteaudio_magic_eraserAudio OverviewflowchartMind Maparrow_downwardJump to bottom