Stratified Prediction-Powered Inference for Hybrid Language Model Evaluation
Best AI papers explained - Podcast tekijän mukaan Enoch H. Kang - Perjantaisin
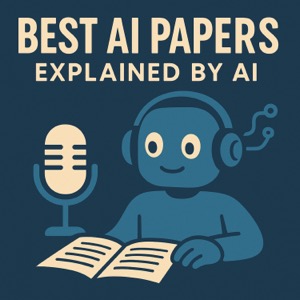
Kategoriat:
This paper introduces Stratified Prediction-Powered Inference (StratPPI), a new method for improving the statistical evaluation of models, particularly Large Language Models (LLMs), which often face costly human annotation bottlenecks. Building on Prediction-Powered Inference (PPI), which combines small amounts of human-labeled data with larger, potentially biased automatic data, StratPPI utilizes data stratification strategies to significantly enhance the accuracy and confidence of model performance estimates. By dividing data into subsets (strata) where automated raters may have different levels of accuracy or bias, StratPPI derives provably valid confidence intervals with tighter margins than unstratified methods. The research demonstrates both theoretically and empirically, through simulations and real-world data experiments, that this stratified approach leads to more reliable and efficient evaluations, especially when automated rater performance varies across different data characteristics.